Intro/Lit Review:
The NFL salary cap is a tool that the league has implemented to prevent one team from spending endless money to get all the top players in the league. This promotes competitiveness and fairness in the league. In an article written by Michael A. Leeds and Sandra Kowalewski in 2011, they look at the NFL salary cap and how that impacts teams in their free agency signings. Past research done by Kowalewski and Leeds (1999) showed that the new collective bargaining agreement during that time resulted in the stars and the veterans benefiting a lot more than the rookies and marginal players. Football is unlike other sports where it is hard to compare different players in different positions. In basketball point guards and shooting guards share similarities but in football comparing players such as quarterbacks and linebackers is hard to do. This can especially be hard in quantifying the impact of them especially when deciding how much to pay each player. In this paper they treated each position separately and ran individual regressions for each position. Specifically for their studies they looked at the skill positions because they are more popular and have the spotlight a lot of the time. They also have an abundance of readily available data because they have the ball a lot of the time.
Their research question for their study was to look at the skill position players and see if the collective bargaining agreement had an impact on the signing of the players. The salary cap is a huge thing in football, and it is what teams must think about when they are filling out their rosters. The salary cap will always be important in football and will always be talked about and researched for teams’ futures.
In this paper to address their research question they found that there were drawbacks to the OLS model so instead they conducted their research “by employing quantile regressions for the 25th and 75th percentile of the conditional wage distribution for 1992 and 1994. This enabled [them] to contrast players who are relatively high-paid with those who are comparatively low-paid. We can thus compare players within each year as well as between years, rather than having to make just one grand comparison” (Leeds and Kowalewski 2001). They used salary data from USA Today findings and got data from quarterbacks, running backs, wide receivers, and tight ends. For their regression their dependent variable was the natural log of the players salary plus bonus payments. They also factored in the number of years played to see how experience impacts salary.
To answer their research question, they used a quantile regression model with the natural log of the players salary plus bonus payments as their dependent variable and saw the impact that different factors of running backs, wide receivers, quarterbacks, and tight ends have on it. In their study, they found that their results were similar to that of Kowalewski and Leeds (1999) in that when determining a players pay, performance was a much more important factor. That before the new agreement players were rewarded more for their position than how they actually played. They found that “the new collective bargaining agreement dramatically changed the criteria by which teams rewarded their players, particularly at lower levels of pay” (Leeds and Kowalewski 2001). They also found that players could increase their pay for increases in passing yardage for quarterbacks, rushing yardage for running backs, receiving yardage for tight ends, and number of receptions for wide receivers.
In a study done by Andrew Larsen, Aju J. Fenn, and Erin Leanne Spenner in 2006, they looked at competitive balance in the NFL and how free agency and the salary cap impacted that. There has been research done relating to fan interest if their team is good or bad. We see that when a team is good, fans want to come to the games but when they are bad fans lose interest and don’t want to watch. In this paper the authors set out to determine the factors of competitive balance and how the combination of free agency and the salary cap has impacted competitive balance in the NFL. In their paper they break it down into multiple sections, first reviewing literature on competitive balance, then discussing competitive balance, then discussing changes to current methods, then discussing their data and regression model, and finally talking about their results.
Their research question is how impactful the salary cap and free agency has been on competitive balance. The salary cap is there to help teams be competitive. If one team could spend all the money that they wanted and sign all the star players, then the league would not be competitive. That one team would win every game and the season would essentially be written before it even started. Fans would not want to come watch and ratings would fall.
In this paper they used the deviation of the HHI to help measure the competitive balance. The then use this model for their study: dHHIt = f(FA/SAL, EXPANt, STRIKE1, STRIKE2, STRIKE3, PLAYER TALENTt, SCHEDULE LENGTH, PLAYOFF SPOTS, RELOCATE, NEW STADIUMS). They used HHI and a model to help answer their question.
Going back to their original question they found that with the addition of free agency, the competitiveness of the NFL has increased. As they describe this makes sense because if a team is no longer allowed to sign all the players there is more of a spread in talent across the league. The league has become more competitive with the addition of free agency and the salary cap. They also tested the impact of different factors on competitive balance. They found that a 14-game season was more competitively balanced than a 16-game season. They also found that the construction of new stadiums affects the competitive balance of the league.
An article by Frank A. Scott, James E. Long, and Ken Somppi looks at NFL free agency and the NFLPA claims that NFL free agency will not work because “owners lack the financial incentive to win that would induce them to bid on free agents” (Scott and Long and Somppi 1983). They claim that since the way that the revenues are split up in the NFL that could limit the financial incentive to win games. A claim for that was that since teams that consistently performed poor had the similar revenues and financials of those that consistently won, what was the incentive for the poor performing teams to get better. In this paper they look at how efficient free agency really is. They look at player mobility. They compare the actions in the NBA of those in the MLB and NBA. They then try to answer the question of if NFL team owners “lack the incentive to win that would induce them to bid on free agents” (Scott and Long and Somppi 1983).
Their research question is does a lack of financial incentive impact NFL owners’ willingness to bid in free agency. At the end of the day the NFL is a business and is there to make money. Many times, the owners have similar mindsets where if they are making money that is all they care about. This can impact NFL free agent players as there is not a bidding war for that player so they are not getting as much money as they would. It can also hurt the competitiveness of the league as star players are not being distributed throughout the league
To answer their question, they use an OLS model. The variables that they include in the model are total revenue, win percentage, income, population, seating capacity of the stadium, and number of professional sport franchises in that city. From their results they found out that team revenue is significantly related to a teams win lost record. That NFL owners do have a financial incentive to win. And because they have an incentive to win, they have an incentive to bid on free agents that would help them win games.
Data and Methodology:
To gather my data, I collected from different sources. I wanted to gather both NFL team spending over the past 10 seasons and the win percentages of the teams each season over the past 10 seasons. To get the salary cap and spending data I collected from Spotrac. To gather the win percentages of the teams I collected from NFL.com. After collecting that data in an excel sheet I then I put it into R where I cleaned and organized it.
The variables in my data set were year, yearly rank of salary space among all teams, team, win percentage, number of players signed, average age, active salary, dead cap, total cap, and cap space. Due to salary cap figures being in the hundreds of millions I divided all the salaries by 1,000,000 and put them in terms of millions. I also multiplied the win percentage by 100 to turn it into percentages instead of decimal form.
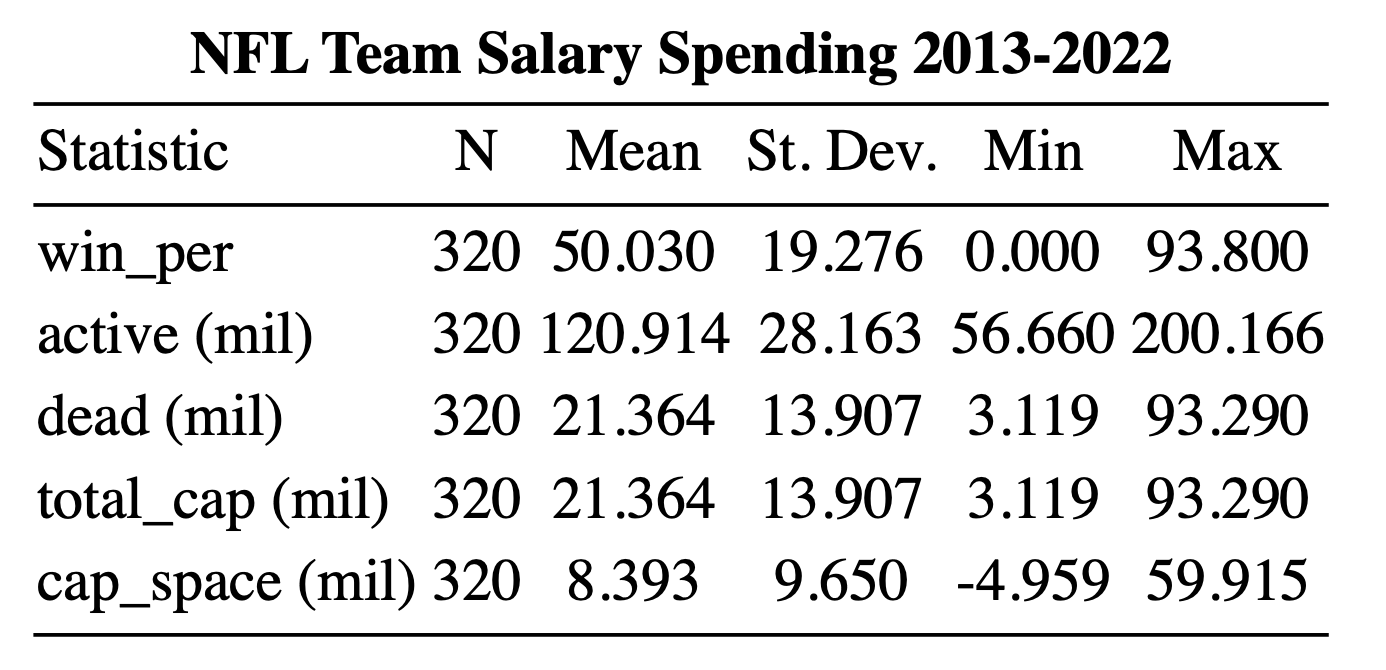
The graphic above shows summary statistics for the variables that I will be using in my model. In general teams used up most of their cap space with only an average of 8.393 million dollars being left over in cap space. Some teams did not use much of their cap space with a team having 59.915 million dollars in cap space. Some teams went over the cap space limit with a team having -4.959 million dollars in cap space.
The data will help me answer my research question because I want to see if spending more leads to a higher win percentage. I want to see if spending leads to more wins and if there is an incentive for teams to spend. I want to also see if there is competitive balance in the league where teams are spending their money and cap space. I want to compare it year by year. To answer my questions, I will be running a regression and creating visuals.
Results and Discussion:
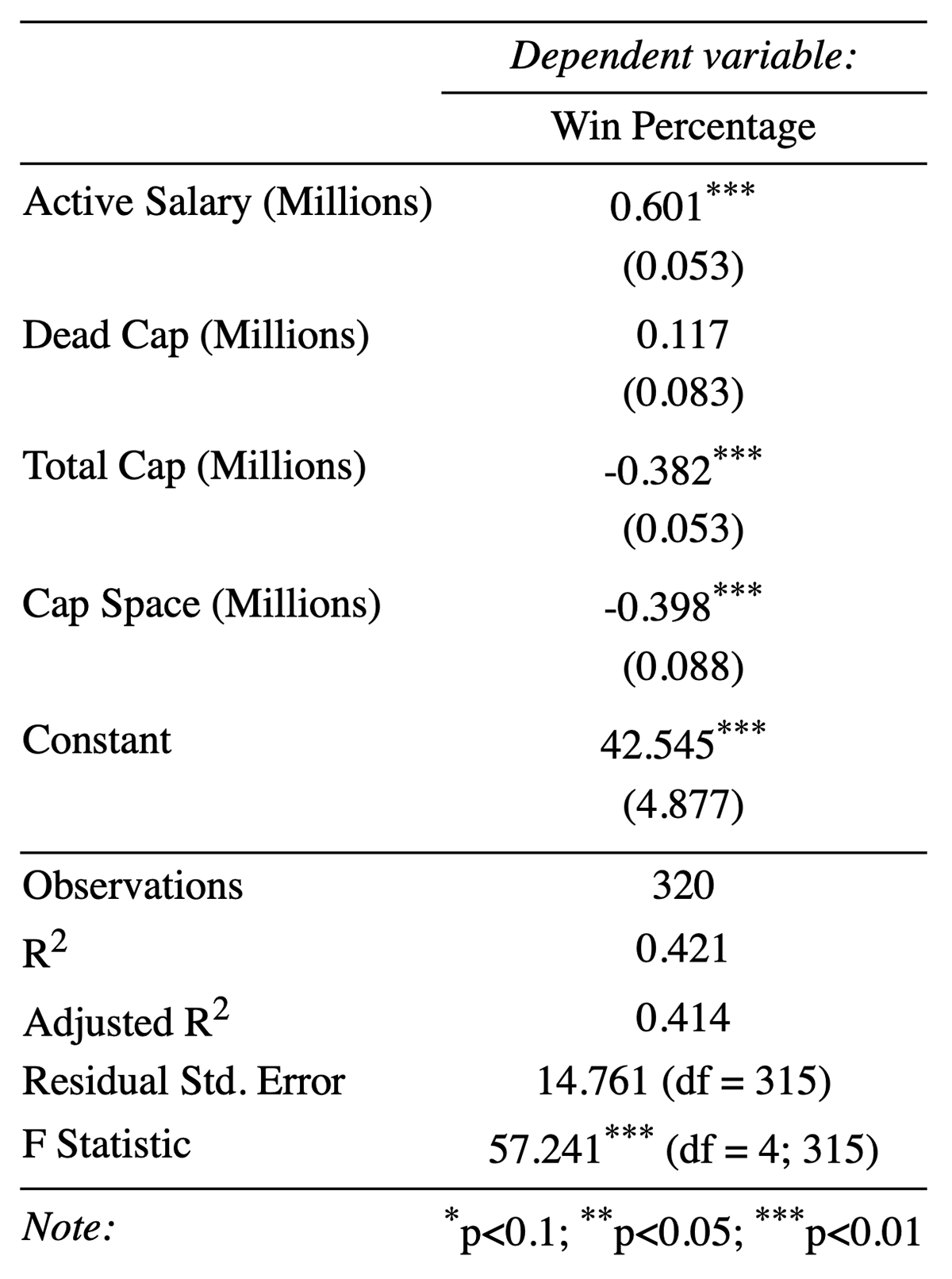
The diagram above shows the regression results from the model. The dependent variable of the model is win percentage and the predictor variables are active salary in millions, dead cap in millions, total cap in millions, and cap space in millions. As can be seen by the above results three of the predictor variables are statistically significant at the 0.01 level. This means that we cannot reject the null hypothesis which suggests that there is a correlation between those predictor variables and win percentage. Those variables are active salary in millions, total cap in millions, and cap space in millions. Looking at the magnitudes of those coefficients, two have a negative relationship and one has a positive relationship. Active salary in millions had a coefficient of 0.601 meaning a 1 million dollar increase in active salary is going to increase win percentage by 0.601. Total cap in millions has a coefficient of -0.382 meaning that a 1 million dollar increase in total cap decreases win percentage by 0.382. Cap space in millions has a coefficient of -0.398 meaning a 1 million dollar increase in cap space decreases win percentage by 0.398.
The results are interesting because of the different relationships between the predictor variables. The results suggest that if teams spend more than their chances of winning goes up. If teams have higher active salaries, which usually means they have some high paid players on their teams, then they have a higher win percentage. On the other side if a team has high cap space, they have a lower win percentage then a team that spends their cap space. These results make sense and what I was expecting because if teams are not spending money on good players, then they have a less chance of winning.
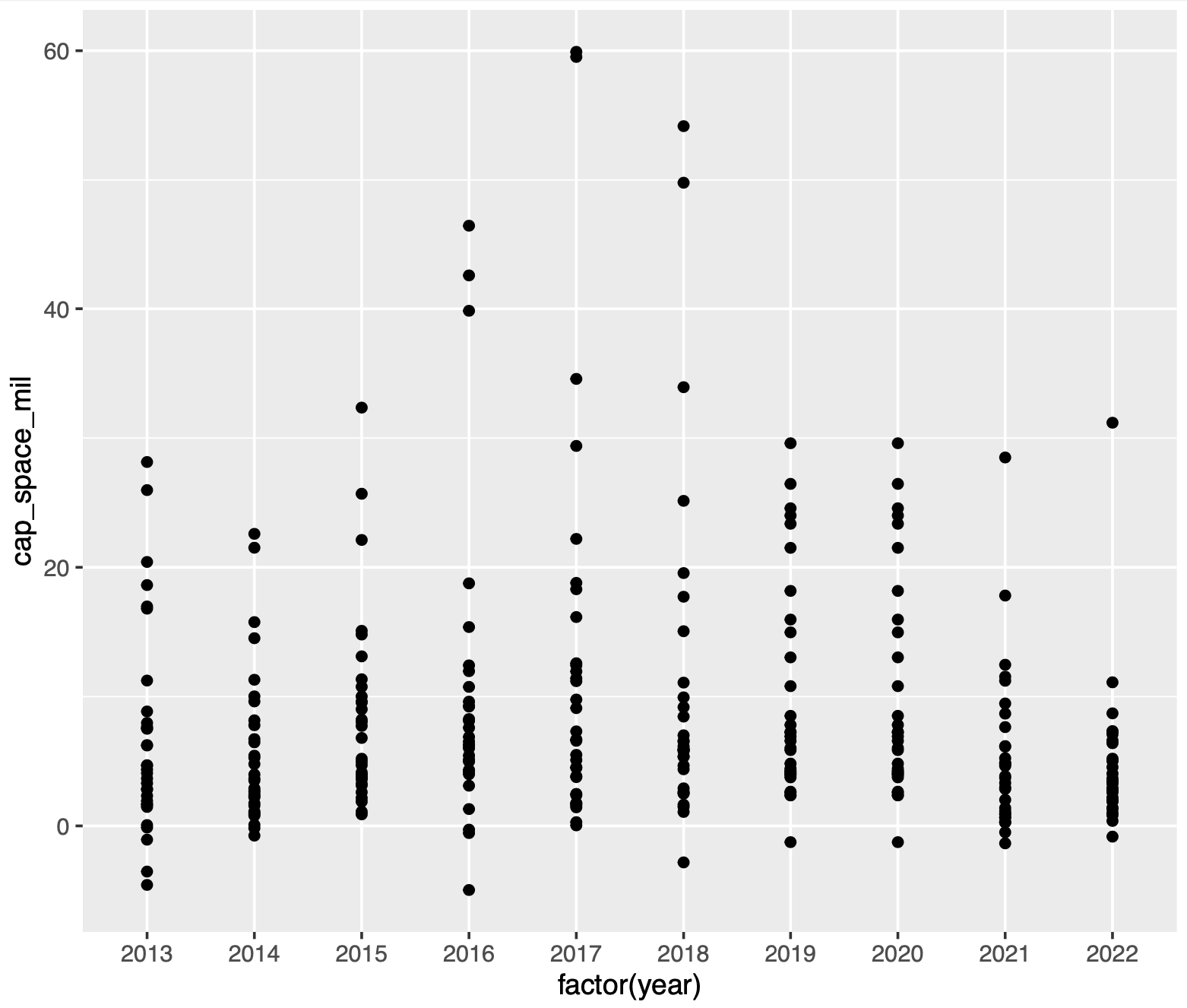
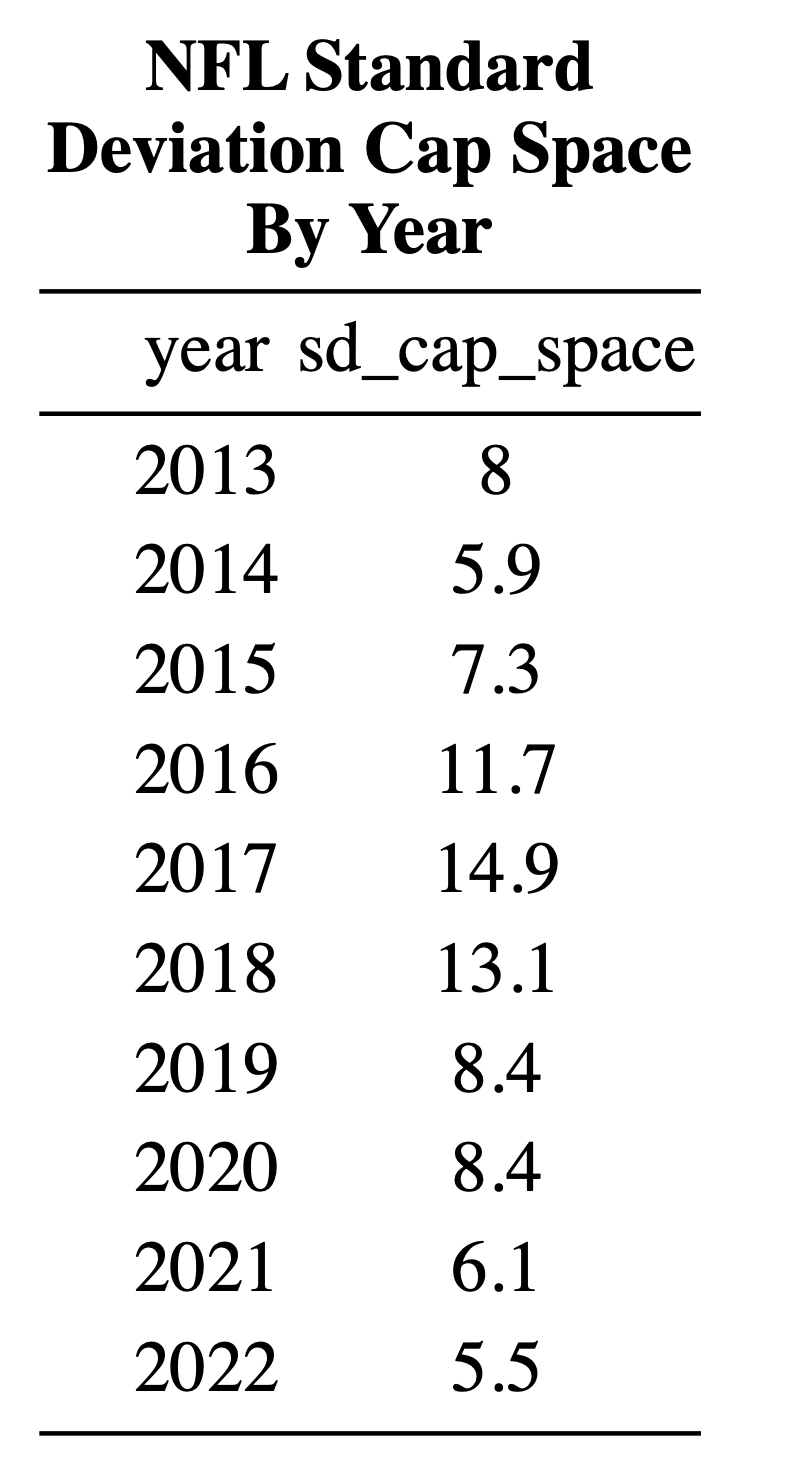
This diagram above on the left shows the distribution of cap space in millions of teams each year over the past 10 years. Some interesting things can be noted here. In the years from around 2015 to 2018 there was more of a spread in salary cap of the teams. Especially in the years 2016, 2017, and 2018 there was more of a spread in salary cap with some very big outliers. Over the past couple years, the spread has been closer together with the exception of one or two outliers. This is interesting especially when comparing it to the regression results. We saw from the regression results that when teams spend more and have less cap space, they increase their win percentage. In certain years there is a wide spread of cap space with some huge outliers. This can be seen by the table on the right. This table shows the standard deviations of cap space for teams every year. Standard deviation is a number that measures the spread and variance of a set of numbers. As can be seen on the table, the biggest standard deviations were in the years 2017 (14.9 standard deviations) and in 2018 (13.1 standard deviations). We can also see this on the graph with the biggest spreads being in those years especially with the big outliers.
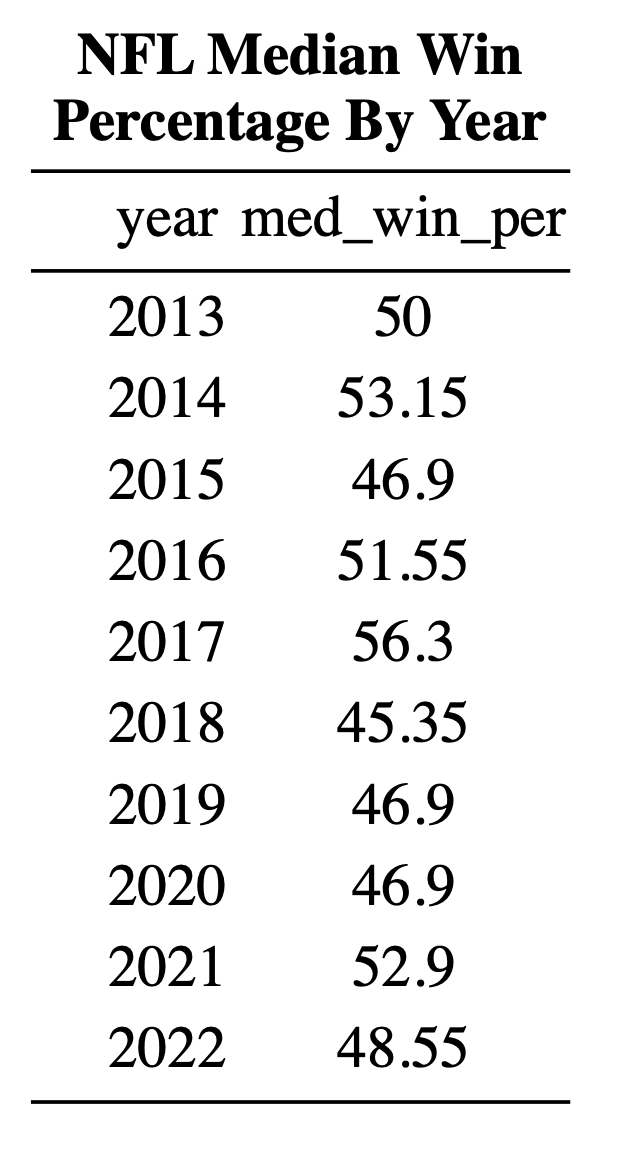
The table above shows the median win percentage of teams every year over the past 10 years. Every year the average win percentage is 50% because each team plays the exact same number of games. The median win percentages can be different based on the competitiveness of the teams in the leagues. If the median win percentage is closer to 50%, that means that the league was more competitive that year. From the table we can see that the least competitive years were 2017 and 2018. If we compare this to the cap space data, we can see that the years that are the least competitive (2017 and 2018) match up exactly with the years that there is the biggest spread of cap space. This suggests that if teams aren’t spending the same amount of money, then it is less likely to be competitive.
Conclusion:
Does a salary cap have an impact on the competitive balance in the NFL? Is there an incentive for teams to spend in order to win? Through looking at NFL team spending data along with the win percentages of those teams over the past 10 years I was able to look at the impact of the salary cap on competitive balance. I created a regression with win percentage as the dependent variable and salary cap figures as the predictors. Median win percentages along with the spread of cap space was also looked at to help solve the main question.
Through the models and tests there appears to be a correlation between teams spending and having higher win percentages. When teams use up their cap space and spend on players, their chances to win increase. Also, when all teams spend similar amounts there is more competitive balance in the league. These results are important when we look at the impact of the salary cap on the league. When used right, the salary cap makes the league more competitive. Although football is a sport that has a salary cap, the results could help add to the argument of adding a salary cap to all leagues, such as baseball for example, to make the league more competitive. In football there are rules and regulations that make teams spend a certain amount of the cap. The results from this study show why that is so important and why the league needs to make sure that all teams spend around the same amounts. The salary cap incentivizes teams to spend as it has proven to increase their chances of winning. The NFL salary cap currently helps keep the league competitive and it will continue to do that for as long as it is in place.
Works Cited:
Larsen, Andrew, et al. “The Impact of Free Agency and the Salary Cap on Competitive Balance in the National Football League.” Journal of Sports Economics, vol. 7, no. 4, 2006, pp. 374–390., https://doi.org/10.1177/1527002505279345.
Leeds, Michael A., and Sandra Kowalewski. “Winner Take All in the NFL.” Journal of Sports Economics, vol. 2, no. 3, 2001, pp. 244–256., https://doi.org/10.1177/152700250100200304.
“NFL Salary Cap Tracker.” Spotrac.com, https://www.spotrac.com/nfl/cap/2022/.
“Official Site of the National Football League.” NFL.com, https://www.nfl.com/standings/league/2022/reg.
Scott, Frank A., et al. “Free Agency, Owner Incentives, and the National Football League Players Association.” Journal of Labor Research, vol. 4, no. 3, 1983, pp. 257–264., https://doi.org/10.1007/bf02685211.